Big Data analytics is different from classic data analysis particularly because of the volume, velocity, and variety of the data being processed. To address the diverse requirements for performing analysis on Big Data, a step-by-step framework is needed. A methodology is required to organize the activities and tasks involved with acquiring, processing, analyzing, and re-purposing data. In this article, we will discuss the Big data analytics life cycle. This cycle organizes and manages the tasks and activities associated with the analysis of Big Data.
Life Cycle of Big Data Analytics
In order to provide a methodology to organize the work and deliver clear insights from Big Data, there is a cycle with different stages. All the stages of the Big Data life cycle are related to each other.
Business Understanding or Discovery
This is the first phase of the Big Data analytics life cycle. Here, the team learns the business domain, along with the relevant history of the organization. For instance, it learns whether the business unit has carried out similar projects in the past from which they can learn. The team assesses the resources available in terms of people, technology, time, and data to support the project. Activities in this phase consist of framing the business problem as an analytics challenge. This phase, particularly, tries to understand the project objectives and needs from a business viewpoint. Further, it converts this knowledge into a data mining problem definition. A plan is designed to achieve the objectives. Big Data analytics life cycle must begin with a well-defined business case. This is because, it presents a clear understanding of the justification, motivation, and goals of carrying out the analysis.
Data Identification or Understanding
Identifying diverse data sources increases the chances of finding hidden patterns or links. For example, to provide insight, it can be profitable to identify as many types of related data sources as possible. This should be done, especially when it is unclear what exactly to look for.
On the basis of the scope of business analysis along with the nature of the business problems, the required datasets, and their sources can be internal as well as external to the business.
The data understanding phase starts initially with data collection. It continues with activities in order to get familiar with the data. It proceeds further identifying data quality problems and uncovering first insights into the data.
Data Preparation
This is the third stage of the Big Data analytics life cycle. The data preparation stage covers all activities to construct the final data set from the initial raw data. Data preparation tasks are mostly done multiple times, but not in any particular order. Tasks done consists of tabling, recording, and attribute selection. It also comprises cleaning and transformation of data for modeling tools.
Model Building
In this stage, the team prepares datasets for testing, training, and production. Additionally, the team also builds and executes models on the basis of work done in the previous phase. The team also considers whether its existing tools will be enough to run the models, or if a more robust environment for executing models is in need.
Evaluation
The team, along with major stakeholders, determines if the results of the project are a success or a failure on the basis of the Phase 1 criterion. The team should list key findings, quantify the business value. It should also prepare a narrative that summarizes and conveys findings to stakeholders.
At this stage of the Big Data analytics life cycle, you have built a model(s). It appears to have high quality, from a data analysis perspective. Before proceeding to the final phase, it is important to evaluate the model thoroughly. Reviewing the steps executed to construct the model, to ensure it achieves the business goals, should also be done.
A key purpose is to find out if there are some business issues that have not been considered enough. At the end of this phase of the big data life cycle, reaching a decision on the use of data mining is a probable outcome.
Deployment
This is the final stage of the Big Data analytics life cycle. In this stage, the team delivers final reports, briefings, code, as well as technical documents. The creation of the model is usually not the end of the phase. Depending on the needs, the deployment phase can be as simple as getting a report. Also, it might be as complex as implementing a repeatable data scoring or data mining process.
In addition, the team may also run a pilot study or project to apply the models in a practical environment.
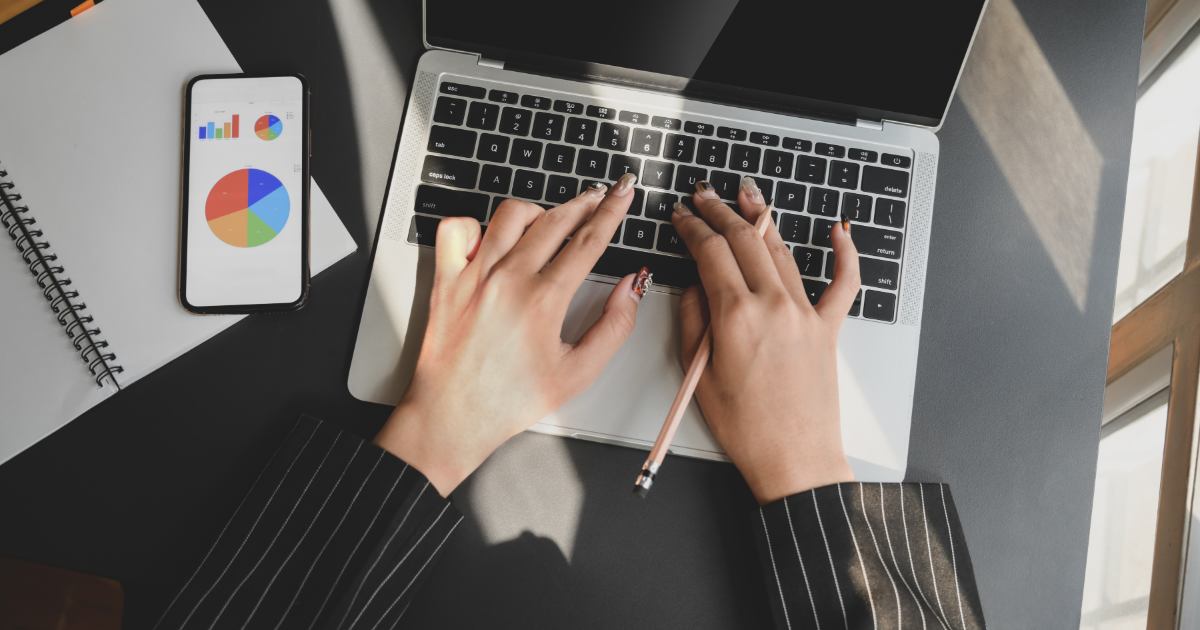
All you need to know about Big Data
Introduction to Big Data | Career Options after Big Data |
4 V’s of Big Data | Big Data for Business Growth |
Uses of Big Data | Benefits of Big Data |
Demerits of Big Data | Salary after Big Data Courses |
Learn Big Data
Top 7 Big Data University/ Colleges in India | Top 7 Training Institutes of Big Data |
Top 7 Online Big Data Programs | Top 7 Certification Courses of Big Data |
Learn Big Data with WAC
Big Data Webinars | Big Data Workshops |
Big Data Summer Training | Big Data One-on-One Training |
Big Data Online Summer Training | Big Data Recorded Training |
Other Skills in Demand
Artificial Intelligence | Data Science |
Digital Marketing | Business Analytics |
Big Data | Internet of Things |
Python Programming | Robotics & Embedded System |
Android App Development | Machine Learning |